A˛C˛ Magazine May 00 Vol. 3 No. 5
pgs. 13-16
Process
Simulation of the Future
by
Jon Paul Van Buskirk, MSChE
Quality Monitoring & Control
Abstract
Computer aided process simulation has
been increasingly popular in the last four decades.
Advances in hardware and software applications driven by the demand of
personal computing has had a positive influence on existing technologies.
The need for both steady state and dynamic simulators in the chemical
industry becomes greater as environmental, quality and safety issues grow with
the expanding industries. Companies are beginning to recognize the benefits that
simulators can provide including research and development, plant design and
retrofitting, and plant operations. Coupled
with modeling, control and optimization, simulation can enable companies to make
better engineering and business decisions in both plant design and operations.
As processes become more complex,
incorporating varying degrees of automation, there is an increasing need in
design and operations using simulators for process understanding.
Modern analysis usually involves mathematical modeling either on a bench
or pilot scale prior to a commercial plant.
Two broad classifications include steady state and dynamic models.
The attention to detail requires a solution based on the accuracy of the
basic data obtained from scaled-down studies; therefore, steady state and
dynamic simulators are highly effective when actual process data is used in
physical property prediction, mass and energy balances, kinetics,
transportation, etc. Process
simulation leads to a deeper understanding of the internal mechanisms and
characteristics of the process.
|
Figure 1:
A Chemical Plant showing the diversity of equipment types
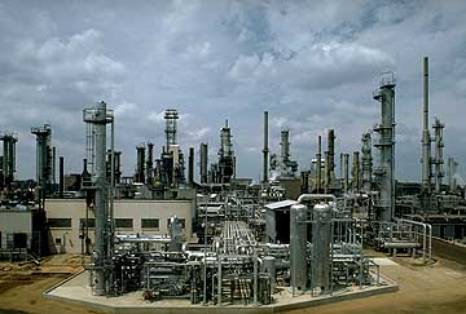
Overview
The Chemical Industry is diverse and
requires several desktop tools for process understanding and improvement.
Figure 1 shows the vast array and number of equipment in a common
chemical process. Equipment is
normally modeled on an individual basis or groups of common functionality.
The number of model types and complexity is also diverse and modeling
methods can range from a first principle approach to data intensive methods such
as genetic algorithms or artificial neural nets.
Data systems can range into the tens of thousands of variables and
modeling methods based on this data is ultimately required to adequately
represent these systems. A model
representation is required for operations, control and optimization of the
process. Modeling benefits include enhanced quality, safety,
environmental, and economic controls.
This article reviews several case
studies leading to process improvement. These
case studies are in reference to actual field applications and include:
|
1.
Steady-State Process Simulation
2.
Dynamic Process Simulation
3.
Detailed
Equipment Design
4.
Modified
Iterative Measurement Test Method
5.
Economic Optimization
Various modeling methods are used in
these Case Studies. In all cases,
actual data is used as the basis for modeling.
This approach has proven to be the most reliable in all applications.
Any industry that uses data can benefit from this approach.
The advantage of this method is that a considerable amount of time can be
saved when data is available for modeling.
The ultimate requirement is that the model adequately represents the
actual system for prediction of future events.
Using actual data for this modeling basis will provide the most robust
results for these predictions.
Case
Study 1:
Facilities Design using a Steady-State Simulator:
A fundamental approach is required for
new facilities design since data is not available.
In the process industries, a simulator is used for mass, momentum, and
energy balances and for chemical-physical property generation.
The fluid components are selected, and the equipment and streams laid out
in a flow sheet fashion. From this
information, the simulator solves for the process conditions in a sequential
method. The results provide the
information necessary for equipment sizing, costing, predictive maintenance and
optimization. Figures 2 and 3 are
typical depictions of process flow sheets by the steady-state simulator from
Design II for Windows™. Figure 2
depicts a simulator for rigorous process design of a refinery. Figure 3 is an
example of a debutanizer column design. One
objective of a simulation may be to design and optimize process operations to
maximize energy savings.
|
Figure 2:
Depicts a simulator for rigorous process design of a refinery
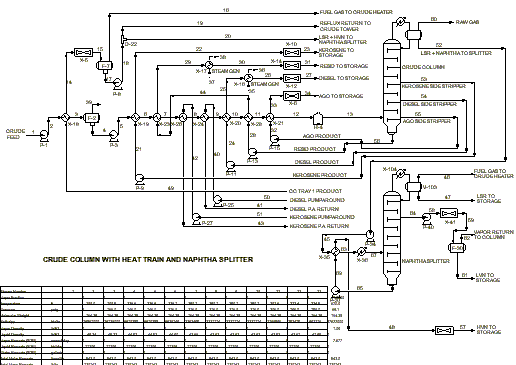
Figure 3:
An example of a debutanizer column design
Case
Study 2:
Facilities Design using a Dynamic Simulator:
Dynamic
transitions in chemical processes cannot be accurately modeled using a
steady-state simulator. For all practical purposes, a dynamic simulator integrated
with a full physics package based on a time dependent method should be used for
transitional data analysis. As with
the steady-state simulator dynamics must include all physical/chemical property
calculations with a flow sheet generator interface. Figure 4 is an example of a simplified flow sheet of a
reactor system the utilizing QMC DYFLO Program for reactor kinetics.
The model is in a dynamic time domain and can also be used for tanks,
pumps, compressors/expanders, heat exchangers, pipes & valves, control
valves, controllers, reactors/combustors, distillation, etc.
The technology has been successfully used in chemical processes for over
30 years with many benefits for system analysis and robust methods for actual
operational evaluation of steady-state and transitional conditions.
|
Figure 4:
An example of a simplified flow sheet of a reactor system
utilizing the
QMC DYFLO for reactor kinetics
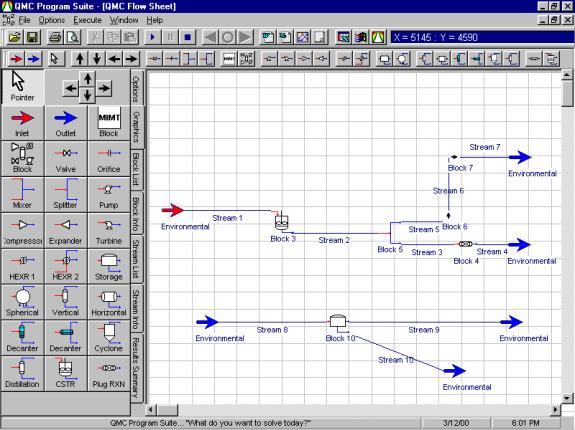
Case
Study 3:
Facilities Design using Detailed Sizing Programs:
From steady-state and/or dynamic
simulation, a detailed design of equipment can be reviewed and verified. In the Chemical Industry, this equipment includes piping,
control valves, safety valves, flow meters, pumps, compressors, heat exchangers,
decanters, gas-liquid separation vessels, etc.
Figure 5 provides the results of a control valve sizing procedure.
Depicted is the control valve flow coefficient, termed CV, versus flow
rate. Also shown is the limit of
flows for this control valve system. Figure
6 is a detailed design program to size a Counterflow Shell and Tube heat
exchanger. Detailed equipment design typically gives thorough results,
i.e. mechanical design, stress analysis, etc., for final design generating spec
sheets for the vendor. |
Figure 5:
Results of a control valve sizing procedure
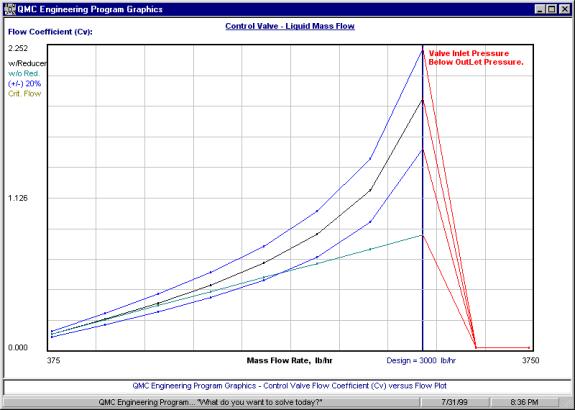
Figure 6:
A detailed design program to size a Counterflow Shell and Tube
heat
exchanger
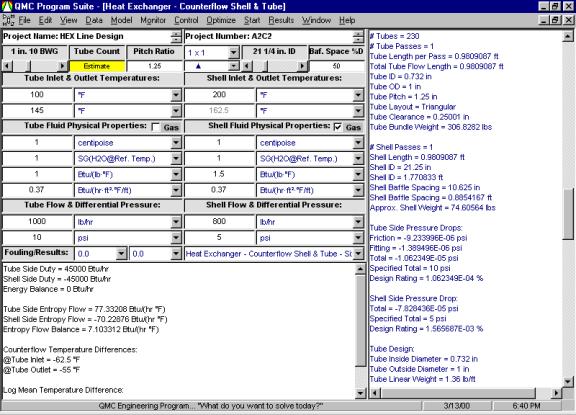
Case
Study 4:
Modified Iterative
Measurement Test Method:
An
enhancement to simulation in order to determine the measured data accuracy is
the Modified Iterative Measurement Test Method (Serth and Heenan, AIChE Journal,
32 p. 733, 1986). The QMC MIMT
Program solves the linear and non-liner
data-reconciliation/gross-error-detection problem.
For a given set of measured data, the program generates a reconciled data
set, i.e., one, which is consistent with all applicable constraints, such as
material balance or model based requirements.
It also generates a list of measurements, which are suspected of being
grossly in error, as well as estimates of the correct values of the suspect
measurements. Typical applications
include total material balances, temperatures, pressures, etc. for chemical
processes or plants, steam-metering systems in plants and refineries, and
natural gas distribution systems. However,
the program is applicable to any data set for which the measured variables are
related to one another by a system of linear or non-linear algebraic equations.
Complex networks of pipes, tanks and process components are shown in
Figure 7.
|
Figure
7:
Complex networks of pipes, tanks
and process components
Case
Study 5:
Economic Optimization:
Economic reporting provides a procedure
to monitor and optimize production costs. The
steps are: a) Perform economic balance, b) Model economics using plant data, and
c) Minimize production costs using a model optimizer. Economic optimization and reporting can run in on-line and
off-line modes.
The economic balance can be done using
spreadsheet functions or a transform calculation program.
The transform calculations should use both fixed and variable costs for a
more complete economic evaluation.
This procedure is shown in Figure 8.
Provided in this figure are the Plant data, calculated economic
variables, and associated economic equations.
Results are saved to a spreadsheet for future analysis.
For this example a small part of the
total process, a reactor system, is used for economic analysis.
This approach allows focus to different operating areas and allows for
localized optimization of individual unit operations. A global approach will help select which areas of the plant
will provide the major profit improvement.
The global approach will also provide a monitoring check on realized
production cost savings.
Figure
8:
Economic Calculations using a
Plant Data Set
|
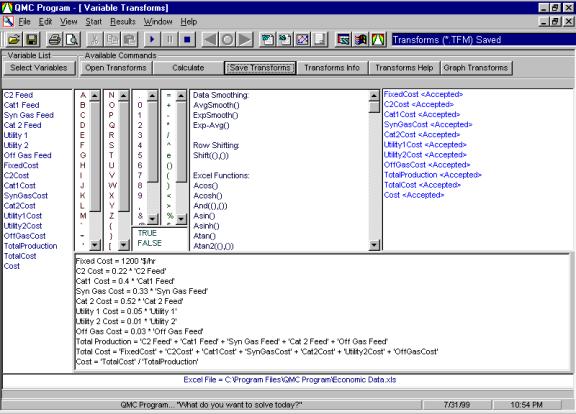
The (sorted) cost per hour is given in
Figure 9. As shown on this graph, a
wide range of operating costs exists. This
is due to a wide range in production rates and the effects of fixed and utility
costs. For this process, it is best
to maximize production rates to minimize product costs.
A model of the cost can include
applicable process data such as pressures, temperatures, flows, and
concentrations. The model can then
be adjusted to minimize the cost with respect to these variables when used as
system set points. The results of
this modeling procedure are also shown in Figure 9 (where Ya are the
actual values and Yp
are the model-predicted values for cost per pound of production.)
Figure
9: Results of a modeling procedure
|
With this model, many methods are
available for optimization. This
provides the set points that minimize costs.
This can be done for both on-line and off-line applications. In this case, the optimum occurs at maximum production rates.
However, this maximum production may be limited by several factors, such
as hardware, raw materials availability, or off-site limitations.
Therefore, a constrained optimization algorithm should be utilized.
Other modeling methods to be considered
include genetic algorithms, neural net, evolutionary, etc., or simulation
methods adapted towards the respective industry.
The modeling technique selected should come with an optimizer that can
handle a wide range of applications. The
maximum benefits of modeling are when an optimization procedure can be utilized.
Conclusion:
This article reviewed several case
studies involving design, simulation, data reconciliation and optimization. These technologies provide a basis leading to process
improvement. These case studies are
in reference to actual field applications and include: Steady-State Process Simulation,
Dynamic Process Simulation, Detailed Equipment Design, Modified
Iterative Measurement Test Method and Economic Optimization.
It was also shown how simulation,
modeling and optimization methods work together in a unified approach to design
and improve any process. When these
methods are used for auditing, control, and optimization improvements, a
quality, safety, environmental, and economic advantage can be obtained by
operating at peak performance levels.
The applications of these techniques are
not limited to the process industries. They
can be adapted to any industry that has data systems including contamination
control and critical cleaning. Implementation
will provide a definite technology advantage, and promote a logical and
systematic approach for continuous improvement.
|
_________________________________________
Jon
Paul Van Buskirk is a Principal with Quality Monitoring & Control.
He has experience in refineries, petrochemical and polymer industries and
may be reached at QMC@QMC.net. |
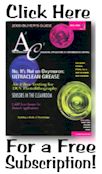
|